Finally, our approach helps us computationally identify several drug combinations for the treatment of hypertension. Our network-based model reveals that ethacrynic acid has the highest likelihood for validating combination with hydrochlorothiazide to treat hypertension Supplementary Table 1 , representing a potential drug combination.
Guanfacine, an approved alpha-2 adrenoreceptor inhibitor to treat hypertension and attention deficit hyperactivity disorder, is predicted to have a high likelihood when used together with hydrochlorothiazide Supplementary Table 1 , representing another potential drug combination for the treatment of hypertension.
In total, we computationally identified potential combinations involving 65 hypertensive drugs with Complementary Exposure to the hypertension disease module Supplementary Data 5. In addition, we provide an exhaustive list of predicted drug combinations involving non-hypertensive drugs with Complementary Exposure and potential adverse drug interactions involving drug pairs with Overlapping Exposure to the hypertension disease module Supplementary Data 5 , offering a potential virtual hypertensive drug combination database for future experimental validation and prospective clinical trials.
Taken together, the network-based models developed here offer a powerful tool to identify efficacious drug combinations for the treatment of hypertension. Combination therapies offer widespread well-documented advantages in the treatment of complex diseases. Here, we demonstrated that a network-based methodology that identifies the relative network configuration of drug—target modules with respect to the disease module can help prioritize potentially efficacious pairwise drug combinations for both hypertension and cancer.
Specifically, our interactome-based approach offers a network-level view of therapeutic combinations in terms of comparative efficacy and adverse interactions. Our key finding is that a drug combination is therapeutically effective only if it follows a specific network topological relationship to the disease module, as captured by the Complementary Exposure pattern.
Somewhat surprisingly, if we are searching for therapeutically synergistic combinations, the two drug—target modules must not only overlap with the disease module, but also need to be separated in the human interactome without overlapping toxicities Here we documented the predictive power of Complementary Exposure in two complex diseases hypertension and cancer based on known drug combination data from publicly available databases.
Therefore, future work is needed to explore the generalizability of our findings to other diseases. Drug pairs with Complementary Exposure are expected to have either therapeutic Fig. For example, a recent clinical study has reported that combining amiloride and hydrochlorothiazide Complementary Exposure in Fig.
Altogether, adverse effects can appear independently from the separation of the two drug target modules, occurring significantly in both Overlapping Exposure Supplementary Fig.
Lack of dose-dependent information and precise perturbation effects of disease-causing variants and drug exposure generate a coupled interplay between adverse and therapeutic effects 31 for Complementary Exposure.
Integration of network proximity, high-throughput in vitro or in vivo assays, pharmacokinetics-based mathematical modeling e. In addition, future work is needed to explore the effects of potential data selection bias.
For example, combinations of drugs that target related proteins in the same disease module are more likely to be tested in drug combination clinical trials. Finally, given the lack of large-scale, systematic data on combinations of multiple drugs, in our current study, we limited our exploration on drug pairs only.
Yet, we expect that Complementary Exposure remains an efficient design principle even upon combining multiple drugs. To fight the combinatorial explosion upon inspecting network relationships of multiple drug—target modules with a disease module, theories of signed networks can be of great help, such as structural balance theories 36 , reducing the number of network patterns to investigate in the human interactome.
In addition, advanced network-based link prediction methods rooted in biological principles 37 can help to develop a combined, quantitative score for each predicted drug combination. Eventually, experimental validation and prospective clinical trials must be conducted to verify the network-predicted drug combinations under controlled conditions.
As for the input data, having a more complete human interactome and more complete, systematic drug—target network with well-annotated pharmacokinetics and pharmacodynamics information would improve the performance of the network-based model further.
In summary, our findings suggest that the discovery of efficacious drug combinations could benefit from network-based, rational drug combination screenings, exploring the relationship between drug—target modules and the disease modules via network proximity in the human interactome.
From a translational perspective, the network tools developed here could help develop novel, efficacious combination therapies for multiple complex diseases if broadly applied.
html ; 2 literature-curated PPIs identified by affinity purification followed by affinity-purification mass spectrometry AP-MS , Y2H, and literature-derived low-throughput experiments; 3 binary, physical PPIs derived from protein three-dimensional structures; 4 kinase-substrate interactions by literature-derived low-throughput and high-throughput experiments; and 5 signaling networks by literature-derived low-throughput experiments.
Computationally inferred interactions rooted in evolutionary analysis, gene expression data, and metabolic associations were excluded. The human protein—protein interactome are provided in the Supplementary Data 1.
Drug—target interactions were acquired from the DrugBank database v4. In total, 15, drug—target interactions connecting drugs and unique human targets were built, including drugs that have at least two experimentally validated targets Supplementary Data 2.
In this study, we focused on pairwise drug combinations by assembling the clinical data from the multiple data sources Supplementary Note 3.
Compound name, generic name, or commercial name of each drug was standardized by MeSH and UMLS vocabularies 47 and further transferred to DrugBank ID from the DrugBank database v4. Duplicated drug pairs were removed.
In total, unique pairwise drug combinations connecting drugs were retained Supplementary Data 3. We compiled clinically reported adverse drug—drug interactions DDIs data from the DrugBank database v4.
Here, we focused on adverse drug interactions where each drug has the experimentally validated target information. Compound name, generic name, or commercial name of each drug were standardized by MeSH and UMLS vocabularies 47 and further transferred to DrugBank ID from the DrugBank database v4.
In total, 13, clinically reported adverse DDIs connecting unique drugs were retained Supplementary Data 4. In addition, we collected cardiovascular event-specific adverse DDIs from the TWOSIDE database TWOSIDE includes over , significant associations connecting 59, drug pairs and adverse events In this study, we focused on 4 types of cardiovascular events: arrhythmia MeSH ID: D , heart failure MeSH ID: D , myocardial infarction MeSH ID: D , and high blood pressure MeSH ID: D We downloaded chemical structure information SMILES format from the DrugBank database v4.
If two drug molecules have a and b bits set in their MACCS fragment bit-strings, with c of these bits being set in the fingerprints of both drugs, the Tanimoto coefficient T of a drug—drug pair is defined as:. T is widely used in drug discovery and development 49 , offering a value in the range of zero no bits in common to one all bits are the same.
We calculated the protein sequence similarity S P a, b of two drug targets a and b using the Smith—Waterman algorithm The Smith—Waterman algorithm performs local sequence alignment by comparing segments of all possible lengths and optimizing the similarity measure for determining similar regions between two strings of protein canonical sequences of drug targets.
The overall sequence similarity of the targets binding two drugs A and B is determined by Eq. This condition ensures that for drugs with common targets we do not take pairs into account where a target would be compared to itself. In order to reduce the noise of co-expression analysis, we mapped PCC a, b into the human protein—protein interactome network Supplementary Methods 2 to build a co-expressed protein—protein interactome network as described previously We used three types of the experimentally validated or literature-derived evidences: biological processes BP , molecular function MF , and cellular component CC , excluding annotations inferred computationally.
The semantic comparison of GO annotations offers quantitative ways to compute similarities between genes and gene products.
We computed GO similarity S GO a,b for each pair of drug target-coding genes a and b using a graph-based semantic similarity measure algorithm 52 implemented in an R package, named GOSemSim The overall GO similarity of the drug target-coding genes binding to two drugs A and B was determined by Eq.
Clinical similarities of drug pairs derived from the drug Anatomical Therapeutic Chemical ATC classification systems codes have been commonly used to predict new drug targets The ATC codes for all FDA-approved drugs used in this study were downloaded from the DrugBank database v4.
The kth level drug clinical similarity S k of drugs A and B is defined via the ATC codes as below. where ATC k represents all ATC codes at the k th level. A score S atc A, B is used to define the clinical similarity between drugs A and B:.
where n represents the five levels of ATC codes ranging from 1 to 5. Note that drugs can have multiple ATC codes. For example, nicotine a potent parasympathomimetic stimulant has four different ATC codes: N07BA01, A11HA01, C04AC01, C10AD For a drug with multiple ATC codes, the clinical similarity was computed for each ATC code, and then, the average clinical similarity was used In this section, we compared the introduced network-based separation Eq.
Here, we examined two measures to quantify the overlap between target sets of drug A and drug B:. Supplementary Figs. The target-set overlap is low for most drug pairs, and the majority To investigate the statistical significance of the observed overlaps, we used a hypergeometric model.
The null hypothesis is that drug targets are randomly located from the space of all N protein-coding genes in the human interactome.
The overlap expected for two target sets A and B is then given by. and the P -values for enrichment and depletion e. A network-based separation of a drug pair, A and B, is calculated via Eq. We evaluated four other different distance measures that take into account the path lengths between two drug target sets: a the closest measure, representing the average shortest path length between targets of drug A and the nearest target of the drug A; b the shortest measure, representing the average shortest path length among all targets of drugs; c the kernel measure, down-weighting longer paths via an exponential penalty; d the centre measure, representing the shortest path length among all targets of drugs with the greatest closeness centrality among drug targets.
Given A and B, the set of drug targets for A and B, and d AB , the shortest path length between nodes a and b in the interactome, we define these distance measures as follows:. We integrated disease—gene annotation data from 8 different resources and excluded the duplicated entries Supplementary Note 4.
We annotated all protein-coding genes using gene Entrez ID, chromosomal location, and the official gene symbols from the NCBI database Each cardiovascular event was defined by MeSH and UMLS vocabularies We used area under the receiver operating characteristic ROC curve AUC to evaluate how well the network proximity discriminates FDA-approved or experimentally validated pairwise combinations from random drug pairs.
We counted the true positive rate and false positive rate at different network proximities as thresholds to illustrate the ROC curve. As negative drug pairs are not typically reported in the literature or publicly available databases, we use all unknown drug pairs as negative samples. In addition, we selected the same portion of unknown drug pairs as positive samples to control the size imbalance.
We repeated this procedure times and reported the average AUC values to compare the performance of different approaches. All statistical analyses were performed using the R package v3. Further information on experimental design is available in the Nature Research Reporting Summary linked to this article.
The code for network proximity calculation is available at github. All other codes used in this study are available from the corresponding author upon reasonable request.
The publicly available human protein—protein interactome Supplementary Data 1 , experimentally validated drug—target interactions Supplementary Data 2 , experimentally validated drug combinations Supplementary Data 3 , clinically reported adverse drug—drug interactions Supplementary Data 4 , and network-predicted hypertensive drug combinations Supplementary Data 5 are available in Supplementary Data 1 — 5.
Sun, X. High-throughput methods for combinatorial drug discovery. Article Google Scholar. Jia, J. et al. Mechanisms of drug combinations: interaction and network perspectives. Drug Discov. Article CAS Google Scholar. Ali, M. Trends in the market for antihypertensive drugs.
Giles, T. Efficacy and safety of nebivolol and valsartan as fixed-dose combination in hypertension: a randomised, multicentre study. Lancet , — Crystal, A. Patient-derived models of acquired resistance can identify effective drug combinations for cancer.
Science , — Article ADS CAS Google Scholar. Tan, X. Systematic identification of synergistic drug pairs targeting HIV.
Zheng, W. Drug repurposing screens and synergistic drug-combinations for infectious diseases. Lipinski, C. Navigating chemical space for biology and medicine.
Nature , — Bulusu, K. Modelling of compound combination effects and applications to efficacy and toxicity: state-of-the-art, challenges and perspectives. Today 21 , — Sun, Y. Combining genomic and network characteristics for extended capability in predicting synergistic drugs for cancer.
Bansal, M. A community computational challenge to predict the activity of pairs of compounds. Zimmer, A. Prediction of multidimensional drug dose responses based on measurements of drug pairs. Natl Acad. USA , — Barabasi, A. Menche, J. Disease networks. Uncovering disease—disease relationships through the incomplete interactome.
Science , Guney, E. Network-based in silico drug efficacy screening. Cheng, F. Network-based approach to prediction and population-based validation of in silico drug repurposing. Article ADS Google Scholar. Network medicine: a network-based approach to human disease. Vidal, M. Interactome networks and human disease.
Cell , — Yildirim, M. Drug—target network. Hopkins, A. Network pharmacology: the next paradigm in drug discovery. Article MathSciNet CAS Google Scholar. Network pharmacology. Kalmanti, L. Safety and efficacy of imatinib in CML over a period of 10 years: data from the randomized CML-study IV.
Leukemia 29 , — DeAngelo, D. Phase 1 clinical results with tandutinib MLN , a novel FLT3 antagonist, in patients with acute myelogenous leukemia or high-risk myelodysplastic syndrome: safety, pharmacokinetics, and pharmacodynamics.
Blood , — Polman, C. A randomized, placebo-controlled trial of natalizumab for relapsing multiple sclerosis. Wolf, G. Jia, Y. Overcoming EGFR TM and EGFR CS resistance with mutant-selective allosteric inhibitors.
Brown, M. Effect of amiloride, or amiloride plus hydrochlorothiazide, versus hydrochlorothiazide on glucose tolerance and blood pressure PATHWAY-3 : a parallel-group, double-blind randomised phase 4 trial. Lancet Diabetes Endocrinol.
Beermann, B. Absorption, metabolism, and excretion of hydrochlorothiazide. Han, P. Synergism of hydrochlorothiazide and nitrendipine on reduction of blood pressure and blood pressure variability in spontaneously hypertensive rats. Acta Pharmacol. Maronde, R. Response of thiazide-induced hypokalemia to amiloride.
JAMA , — Greene, J. Putting the patient back together—social medicine, network medicine, and the limits of reductionism. Bibi, Z. Role of cytochrome P in drug interactions.
Cami, A. Pharmacointeraction network models predict unknown drug—drug interactions. PLoS ONE 8 , e Zhao, S. Systems pharmacology of adverse event mitigation by drug combinations.
Tatonetti, N. Data-driven prediction of drug effects and interactions. Facchetti, G. Computing global structural balance in large-scale signed social networks. Istvan, A. Network-based prediction of protein interactions. Rolland, T. A proteome-scale map of the human interactome network.
Rual, J. Towards a proteome-scale map of the human protein-protein interaction network. Law, V. DrugBank 4. Nucleic Acids Res. Zhu, F. Therapeutic target database update a resource for facilitating target-oriented drug discovery.
Hernandez-Boussard, T. The pharmacogenetics and pharmacogenomics knowledge base: accentuating the knowledge. Gaulton, A. ChEMBL: a large-scale bioactivity database for drug discovery. Liu, T. BindingDB: a web-accessible database of experimentally determined protein—ligand binding affinities.
Pawson, A. Apweiler, R. UniProt: the universal protein knowledgebase. Bodenreider, O. The Unified Medical Language System UMLS : integrating biomedical terminology.
Open Babel: an open chemical toolbox. Willett, P. Similarity-based virtual screening using 2D fingerprints. Today 11 , — Smith, T. Identification of common molecular subsequences. Studying tumorigenesis through network evolution and somatic mutational perturbations in the cancer interactome.
Wang, J. A new method to measure the semantic similarity of GO terms. Bioinformatics 23 , — Yu, G. GOSemSim: an R package for measuring semantic similarity among GO terms and gene products. Bioinformatics 26 , — Prediction of polypharmacological profiles of drugs by the integration of chemical, side effect, and therapeutic space.
NCBI Resource Coordinators. Database resources of the National Center for Biotechnology Information. Download references. The authors thank Yifang Ma, Marc Vidal, and Joseph Loscalzo for useful discussions on the manuscript. The authors thank Alice Grishchenko for polishing the figures.
This work was supported by NIH grants PHG and UHG to A. from NHGRI, P01HL to A. from NHLBI, and K99HL and R00HL to F. from NHLBI. Center for Complex Networks Research and Department of Physics, Northeastern University, Boston, MA, , USA. Feixiong Cheng, István A.
Center for Cancer Systems Biology and Department of Cancer Biology, Dana-Farber Cancer Institute, Boston, MA, , USA. Genomic Medicine Institute, Lerner Research Institute, Cleveland Clinic, Cleveland, OH, , USA.
Department of Molecular Medicine, Cleveland Clinic Lerner College of Medicine, Case Western Reserve University, Cleveland, OH, , USA. Case Comprehensive Cancer Center, Case Western Reserve University School of Medicine, Cleveland, OH, , USA. These were the first times this occurred in the life of the mission.
All of the safehold events so far are listed below. Changes were made to the Faraday Cup flight software to alter the behavior of the instrument. Essentially, the instrument now waits longer between scans to reduce the impact of spurious noise that was causing the instrument to lose the solar wind proton peak.
This change may not eliminate the loss of peak tracking issue completely, but it is a significant step in the right direction.
A change was made to the Faraday Cup processing to remove some of the noise that was resulting in higher than expected densities and temperature. The change ignores high energy noise that was resulting in wider than expected velocity distributions. NOAA's Deep Space Climate Observatory DSCOVR became the operational RTSW spacecraft.
It replaced the NASA Advanced Composition Explorer ACE spacecraft, which has been in use since Real-time Solar Wind and Magnetometer data is now available in JSON format for up to the past 7 days from the SWPC Data Service.
These JSON files will automatically include the data from the active RTSW spacecraft. By default, that has been DSCOVR since July 27 at UT. A complete DSCOVR data archive is available at the NOAA National Center for Environmental Information.
Skip to main content. R1 Minor Radio Blackout Impacts. HF Radio: Weak or minor degradation of HF radio communication on sunlit side, occasional loss of radio contact. Navigation: Low-frequency navigation signals degraded for brief intervals.
Real Time Solar Wind. Black background White background Marker Line Hybrid Line 6 hrs Left Y-axis labels Alternating Y-axis labels Show flags. Usage Impacts Details History Data Real-Time Solar Wind RTSW data refers to data from any spacecraft located upwind of Earth, typically orbiting the L1 Lagrange point, that is being tracked by the Real-Time Solar Wind Network of tracking stations.
SWPC maintains the ability to instantaneously switch the spacecraft that provides the RTSW data. Maybe the biggest one is, you can get access to all of the RTSW plasma and magnetometer since February As you zoom in to shorter time periods, the resolution of the data displayed will increase automatically.
The highest resolution available can be 1 second magnetometer and 20 second thermal plasma data. You can view data from the operational spacecraft or choose between DSCOVR and ACE.
The geomagnetic K and A indices can also be plotted. Tabs along the bottom of the plot allow different default plots to be chosen. These include data ranges of 2 hours up to ~20 years and displays with only Magnetometer, only Solar Wind Plasma, or a combination of both as well as other features described below.
Display of data values: When mousing over the data or 'tapping' with a mobile device , data values are displayed within the graph.
Hooper Holmes Inc Forecast, Short-Term " HH" Stock Price Prognosis for Next Days · HH Forecast, Long-Term Price Predictions for Next Months and Year: , HH where Ho = H-1(H - HS)H-. Best linear unbiased pr ediction. We wr-ish X'R-'X X'R-'Z X'R'Be X'R y. Z'R-'X Z'R-1Z + G-1 Z'R-'B. - GBu v4 + BjA _ Z'R Predictions of non-landmark gene expression showing a spatial expression along the A–P and D–V axes and b a stripe pattern according to
X hh prediction - x. Observed values of predictor variable. y. Observed values of response variable. newdata x values for which predictions are calculated Hooper Holmes Inc Forecast, Short-Term " HH" Stock Price Prognosis for Next Days · HH Forecast, Long-Term Price Predictions for Next Months and Year: , HH where Ho = H-1(H - HS)H-. Best linear unbiased pr ediction. We wr-ish X'R-'X X'R-'Z X'R'Be X'R y. Z'R-'X Z'R-1Z + G-1 Z'R-'B. - GBu v4 + BjA _ Z'R Predictions of non-landmark gene expression showing a spatial expression along the A–P and D–V axes and b a stripe pattern according to
Latest matches with results Valencia vs Real Madrid. Real Madrid 5 - 1 Valencia. Valencia 1 - 0 Real Madrid. Real Madrid 2 - 0 Valencia.
Real Madrid 1 - 1 Valencia pen Real Madrid 4 - 1 Valencia. Valencia 1 - 2 Real Madrid. Direct matches stats Valencia Real Madrid All direct matches VAL home REA away VAL away REA home. Matches: Statistics of the season All Home Away. Real Madrid.
Table Spain La Liga. All Home Away. Go to the league. Table Champions League. Hugo Duro vs Jude Bellingham. Show schedule for teams Valencia and Real Madrid. Select team to H2H. Select two teams to view direct Team Comparison. H2H today's matches. Head to head H2H top events.
today Minnesota United Columbus Crew. Vitesse Twente. Juventude Internacional. Valencia Real Madrid. Millonarios La Equidad. Deportivo Pereira America de Cali.
Copyright © FcTables. All rights reserved, Contact. Cookies and privacy policy Our partners: Free tips Links. Real Madrid Atletico Madrid. Athletic Bilbao. Real Betis. Real Sociedad. Valencia Las Palmas. Rayo Vallecano. Dozens of ID prediction methods have been published, and both predicted and experimentally derived properties of IDRs, as well as annotations related to their function, are stored in dedicated databases 4.
However, the large variety of available predictors makes it difficult to compare their performance, which can confound biologists wanting to make an informed choice.
To address this issue, the Critical Assessment of Protein Intrinsic Disorder CAID 2 was introduced to benchmark ID and binding predictors on a community-curated dataset of novel proteins obtained from the DisProt database 5.
In CAID, participants submit their implemented prediction software to the organizers, who generate predictions by executing the software on selected protein targets whose disorder annotations were not previously available. Given a new protein sequence, the task of an IDR predictor is to assign a score to each residue for the tendency to be intrinsically disordered at any stage of the protein life.
In CAID, both the accuracy of prediction methods and technical aspects related to software implementation are evaluated. However, accessing the prediction power of the tools is not always possible.
Often, the software is not publicly available, exists solely as a stand-alone executable, or is available as a web server with limitations. Moreover, publicly available methods are not standardized and require informed use, often entailing careful reading of the corresponding publication and interpreting predictors' output.
To address these issues, we present the CAID Prediction Portal, a web server that executes all CAID methods with a single click on a user-defined input sequence.
The server generates a standardized output and facilitates comparing methods, and it produces a consensus prediction that highlights high-confidence disordered regions.
Disordered or binding residues are identified by selecting a threshold on the prediction score. Depending on the type of benchmark, different thresholds can be selected, leading to different results.
To guide the user in selecting the best parameters, the website is accompanied by extended documentation that explains the meaning of the different statistics presented in CAID and provides a brief description of all the methods. While anonymous usage of the CAID Prediction Portal is always permitted, interested users can choose to use an optional log in to recover previous sessions via a private dashboard.
An overview of the CAID Prediction Portal is provided in Figure 1. The CAID Prediction Portal needs to execute many different predictors on the same input sequence, provided by the user.
org that interacts with the scheduler controller of a computing cluster through the Distributed Resource Management Application API DRMAA 6 , a high-level API that provides a standardized interface for submitting and managing jobs on a wide range of cluster systems.
com as a job scheduler for the cluster. The purpose of this implementation is to allow users to submit, monitor and manage jobs on the computing cluster through a friendly web interface which exploits the RESTful API provided by the DRF. We also implemented various management features, such as the ability to stop or delete jobs, and to retrieve the job state, history and outputs for a particular user.
The server provides OAuth 2. When authenticated the user is able to recover previous sessions via a private dashboard. Non-authenticated users are allowed to create new jobs and access the results.
However, the amount of resources available to a single non-authenticated user is more limited, meaning that the number of daily and burst requests allowed is reduced. The DRF back-end is also responsible for managing all the possible jobs that can be submitted to the cluster, the resources to allocate for each specific job e.
CPUs, random access memory , and the dependencies that can be created between different jobs. For the CAID Prediction Portal, we created separate jobs for each of the available predictors, and a few additional jobs for creating input data for some predictors such as PSI-BLAST 7 , HHBlits 8 , SPIDER2 9.
This separation of predictors into different jobs is crucial as it provides flexibility to execute only the predictors of interest and display the results of fast predictors without waiting for others to finish.
The CAID Prediction Portal includes a server dark background , which accepts a protein sequence as input, and a computing cluster pale background , which generates the output, which is available as a table TSV format and rendered in a dynamic feature viewer on the web interface.
io containers to containerize all the predictor software in order to standardize the input and output data, and ensure reproducible results.
By containerizing the software, we can ensure that the software runs consistently across different machines, and most importantly it is not needed to install it manually in each machine. Furthermore, containerizing the predictors enables us to package all the necessary software and dependencies together, making it easier to deploy and update the predictors.
With the creation of the container we also included scripts that are executed before and after the predictor, in order to standardize the input and output of the container, creating an interface with the predictor software.
The input of the predictor is a FASTA file containing multiple sequences, and the predictor is executed on each sequence, producing one output per sequence please note that this should not be confused with the input of the CAID server, which is restricted to a single sequence.
The execution time of the predictor for each sequence is also recorded. Some software present in the CAID Prediction Portal requires additional inputs, such as the results of PSI-BLAST, HHblits, or SPIDER2, to make their predictions. These additional inputs can be created inside the software's container itself, but they can also be provided in most of the cases as an additional parameter.
This ensures that the computation of common inputs is not duplicated, leading to faster and more efficient predictions. com containers because Singularity is designed specifically for high-performance computing environments and has several advantages in the context of computing clusters.
Firstly, Singularity does not require root access, making it easier to deploy and manage in a shared computing environment. Secondly, Singularity is optimized for running scientific workloads, with features such as support for MPI Message Passing Interface and GPUs Graphical Processing Units.
Thirdly, Singularity images can be easily hosted on a variety of storage systems, such as local filesystems, networked file systems, and cloud storage.
To make the container size smaller, some large datasets such as UniRef90 10 , Uniclust30 11 or large machine learning models are mounted inside the container at runtime.
This approach allows the container to access these datasets only when needed, rather than including them in the container itself. However, it is important to note that if these mounts are not created, the script that runs the predictor inside the container will fail with an error, since it will not be able to access the required data.
In order to provide a comparison baseline, we also integrate the AlphaFold-disorder 12 method that infers disorder and binding predictions by exploiting AlphaFold predicted structures available in public databases As the last step of our standardization process, we opted to create individualized tasks for each predictor that can be conveniently executed through the CAID Prediction Portal.
This implementation grants users a heightened level of flexibility in their selection of methods, allowing them to make informed decisions that best suit their specific needs.
Each predictor execution is linked to an API call through the portal's front-end interface, while also remaining compatible with stand-alone usage for batch executions. The API is publicly available and lets third party services request specific predictions on demand.
Full documentation is available on the website. In Table 1 , we reported all methods available in the CAID server along with the corresponding publication when available. These methods are a subset of those evaluated in the second round of the CAID challenge, i.
those for which the authors gave permission or those that were already publicly available and licensed for free use. Given the repertoire of different flavors predicted by the various methods, in the CAID Prediction Portal, we divided them into two broad disorder and binding categories.
Users interested in specific subcategories or flavors are invited to read the description of the methods as reported on the website.
All methods generate predictions from the protein sequence. Some methods require additional input which is generated by helper methods, e. BLAST or HHblits for sequence profiles. In those cases, the additional input is generated once and shared with all dependent methods.
The AlphaFold-disorder 12 method, instead of using the sequence, takes as input the protein structure predicted by AlphaFold. In the CAID Prediction Portal the structure is retrieved directly from the AlphaFoldDB 13 database by searching the UniProtKB accession number.
The server tries to retrieve the accession number by querying the UniProtKB mapping service with the provided sequence encoded with the CRC64 algorithm, and selecting the first result.
If the protein sequence is not present in the UniprotKB, no structure can be downloaded and the predictor will fail to execute. Methods are listed in alphabetical order. The Type column indicates the type of output and the values in parentheses indicate the predictor name suffixes which correspond to different flavors or different implementations.
When available, the corresponding publication is provided along with the corresponding authors. For new methods, authors are those that submitted the method to CAID. The CAID Prediction Portal website allows users to execute the available predictors on a provided protein sequence.
The server can process only one sequence at a time. The predictors that are going to be executed can be configured, with some pre-made settings e. running only disorder, binding or quick predictors , or manually, selecting the predictors of interest. When submitting a new job, the user can also decide to associate a description to the job and an email address that will be used to send a notification when all the predictors will finish executing.
The job name is helpful to attach a text description or just a meaningful identifier to the input sequence, while the user email can be used to receive a notification when the calculation is done.
After the submission, the user will be redirected to the results page. At the top of the page, a header card will be displayed, this contains various information about the execution status of the predictors, along with a control for stopping the jobs still executing, and a button to download all the currently available results in tab-separated values TSV format.
The result page will poll the back-end server to update the status of the jobs that did not finish yet, to retrieve their current status and download the results from the server when available. These results will be used to create and update a feature viewer, to display the outputs of the predictors.
These outputs are all aligned to the protein sequence that was submitted, and they can be of two different types, a binary score and a probability score. The feature viewer offers various controls to manipulate the display of the results.
The predictions can be filtered based on their type disorder or binding , the threshold for the binary score can be changed from the predictor's default to optimized thresholds as provided by CAID.
Optimized thresholds correspond to a selection of metrics reported by the CAID challenge. The optimization strategy depends on the type of metric and validation dataset, those available in the CAID Prediction Portal are described in the website documentation, while we refer to the CAID paper 2 for a full description of all possible benchmarks.
The methods can be sorted based on their performance in CAID, disorder or binding content, or alphabetically based on their names. In the feature viewer, a consensus is also computed with the prediction of the available predictors, divided in the two categories, disordered and binding.
This consensus is calculated as a majority vote of the binary predictions available. The consensus will also be influenced by the chosen threshold.
In order to compare predictions with structural and functional domains, Pfam 43 and Gene3D 44 assignments from the InterProScan 45 output are reported. These annotations are calculated in parallel on a separate job, and shown as separate tracks on the feature viewer when available. While anonymous usage of the CAID Prediction Portal is always permitted, interested users can choose to recover previous sessions via a private dashboard after a login using their ORCID credentials, where all the previously submitted jobs can be accessed.
An anonymous user can recover a previous job by saving its UUID and later use it to access the results again. The CAID Prediction Portal is a valuable resource for researchers and scientists working in the field of protein structure and intrinsic disorder prediction.
By combining state-of-the-art ID and binding prediction methods with the CAID optimization strategy, the portal allows users to calculate and compare different predictions in a single view. Predictions can be dynamically adapted on the fly by choosing different CAID optimization strategies.
For example, the user can focus on precision over recall, or on the contrary, can relax the optimization cutoffs to expand disorder detection. One of the key advantages of the portal is its speed and dynamic nature, as the server displays the results of a method as soon as the calculation is completed.
Additionally, the portal's modular and extensible design makes it easy to add or remove prediction methods at any time, providing maintainers with the flexibility to adapt to new developments in the field. Finally, all methods are standardized and their output is made available in the same format.
The CAID section of the portal provides benchmarking results and statistics that can guide users in the evaluation of the performance of the predictors. This information is particularly useful for researchers who are looking to improve their methods and algorithms. Moreover, the CAID Prediction Server is integrated into the OpenEBench 46 infrastructure for community benchmarking experiments of computational methods in the life sciences, which displays the results of various CAID editions in a dedicated section.
This integration allows for the prediction output generated by the portal to be used in generating assessment results, thereby facilitating a transition from a timeframe-based challenge as was the case for CAID rounds 1 and 2 into a continuous assessment.
Last but not least, the CAID portal will help inform and improve the selection ID predictors available in the MobiDB database 47 for large-scale annotation of ID in proteins. The latter is the main source of ID data for core data resources such as InterPro 48 and UniProtKB Any small improvement in ID prediction performance documented in the CAID Portal therefore has a large potential knock-on effect in improving ID annotations across the known protein universe.
Free Bets are paid as Bet Credits and are available for use upon settlement of bets to value of qualifying deposit. Min odds, bet and payment method exclusions apply.
New customers only. ad Claim now. Diriangén Last Games In the last 10 games Diriangén has won 8 times, they have lost 0 times, and it has ended in a draw 2 times. Win 8 Draw 2 Lose 0. Win 4 Draw 2 Lose 4. Diriangén Over 2. Diriangén Diriangén score 21 goals in the last 10 games.
Scored 21 goals Conceded 6 goals Scored. Scored 18 goals Conceded 16 goals Scored. Diriangén Home Results In the last 10 home games Diriangén has won 7 times, they have lost 1 time, and it has ended in a draw 2 times. Win 7 Draw 2 Lose 1.
Win 5 Draw 2 Lose 3. H2H Date Match League Result Game Odds Bookmaker 1 X 2 1xBet 1. Diriangén Last Results Date Match League Result More Predictions All Games.
Esan Pattaya.
Compositions are generated by combinatorially combining sets of X, Y, and Z elements found in reported HHs. As expected from the combinatorial composition Equation () is used to find those values of X for which the range S(0) ≤ X H.H. Kausch and C. Oudet, 'Progress and challenge in polymer crazing and (H.H.).) +s"(/),(H),t - (How) +(H.H.) so) + COnSt W W One can see that the logCs(s) is a quadratic form of kernel (x, x) 0K(x,.X Probabilistic Methods: X hh prediction
Sudlow, C. Koene RJ, Prizment AE, Blaes A, Konety SH. Author predictiion Author notes Hu authors x hh prediction equally: Aniruddh P. The GPS Mult was compared with previously published polygenic scores with respect to effect size for CAD association. Combined with discrete molecular dynamics DMD [31]coarse-grained approaches [31] — [33] can be used to predict structures as well as folding mechanisms with knowledge-based potentials derived from known structures. | As for the input data, having a more complete human interactome and more complete, systematic drug—target network with well-annotated pharmacokinetics and pharmacodynamics information would improve the performance of the network-based model further. Study design of ASPirin in Reducing Events in the Elderly ASPREE : a randomized, controlled trial. However, we noticed that some genes lost the predictive accuracy compared with the reconstruction accuracy Supplementary Fig. George , S. Protein disorder prediction: implications for structural proteomics. | Hooper Holmes Inc Forecast, Short-Term " HH" Stock Price Prognosis for Next Days · HH Forecast, Long-Term Price Predictions for Next Months and Year: , HH where Ho = H-1(H - HS)H-. Best linear unbiased pr ediction. We wr-ish X'R-'X X'R-'Z X'R'Be X'R y. Z'R-'X Z'R-1Z + G-1 Z'R-'B. - GBu v4 + BjA _ Z'R Predictions of non-landmark gene expression showing a spatial expression along the A–P and D–V axes and b a stripe pattern according to | x=rnorm(20), y=rnorm(20)) autoscout.world <- lm(y ~ x, data=tmp) autoscout.world(autoscout.world). HH documentation built on Aug. 9, , p.m.. Related to autoscout.world in HH HH index x Banner. x. Free Sport Predictions & Betting Tips. Betensured will provide free Prediction, Odds. KK Dinamo Zagreb vs Alkar, 1 HH, ? Luton Town vs Aston X c. 1. Tc. X t jxc,t ю lc,tj. П25ч where xc,t is the trend feature for The. DLSTM prediction has a more continuous prediction (a smoother curve) than CNN | PREDICTION: The administration will focus on the HH jobs numbers (+,) instead of the industry-standard establishment numbers (+98,) Tell us how fast you ran a previous race, and our race time calculator gives you predicted race times for race distances from 5k to miles x. Observed values of predictor variable. y. Observed values of response variable. newdata x values for which predictions are calculated | ![]() |
In Table 1we reported all methods available in x hh prediction CAID predicgion along with the corresponding publication when available. GuoR. GreenE. from NHLBI. Additional predictors in men included grip strength, smoking, BMI and renal function. | The validation datasets were composed of UK Biobank participants separate from those used to train the GPS Mult. g Cross-validation CV study tenfold. Brownbill , L. CPUs, random access memory , and the dependencies that can be created between different jobs. Rood, J. The methods can be sorted based on their performance in CAID, disorder or binding content, or alphabetically based on their names. Article Google Scholar Friedman J, Hastie T, Tibshirani R. | Hooper Holmes Inc Forecast, Short-Term " HH" Stock Price Prognosis for Next Days · HH Forecast, Long-Term Price Predictions for Next Months and Year: , HH where Ho = H-1(H - HS)H-. Best linear unbiased pr ediction. We wr-ish X'R-'X X'R-'Z X'R'Be X'R y. Z'R-'X Z'R-1Z + G-1 Z'R-'B. - GBu v4 + BjA _ Z'R Predictions of non-landmark gene expression showing a spatial expression along the A–P and D–V axes and b a stripe pattern according to | Valencia in La Liga 9 pos (36 points) Real Madrid in La Liga 1 pos (65 points) in Champions League 1 pos (18 points). Predictions: 1: 17%. X: 24%. 2: 58% Predictions of non-landmark gene expression showing a spatial expression along the A–P and D–V axes and b a stripe pattern according to These are very different types of predictions and the distinction between risk and diagnosis is important for reporting prediction studies. X-ray in | Hooper Holmes Inc Forecast, Short-Term " HH" Stock Price Prognosis for Next Days · HH Forecast, Long-Term Price Predictions for Next Months and Year: , HH where Ho = H-1(H - HS)H-. Best linear unbiased pr ediction. We wr-ish X'R-'X X'R-'Z X'R'Be X'R y. Z'R-'X Z'R-1Z + G-1 Z'R-'B. - GBu v4 + BjA _ Z'R Predictions of non-landmark gene expression showing a spatial expression along the A–P and D–V axes and b a stripe pattern according to | ![]() |
AmorT. No deposit promo information Journal peer review predictino Nature Casino free bonus no deposit mobile thanks the anonymous x hh prediction for their predictoon to the peer review of this work. Error bars indicate the standard deviation. Consider, for example, amiloride and hydrochlorothiazide, in the Complementary Exposure P2 in Table 1 class. Additional predictors in men included grip strength, smoking, BMI and renal function. | PubMed PubMed Central Google Scholar Purcell, S. Fanning , M. SPOT-Disorder2: improved protein intrinsic disorder prediction by ensembled deep learning. The outcome data was accompanied by measurement of a wide range of clinically relevant and recognized geriatric potential prediction variables biomedical and social collected near the time of study initiation. The Vfold server contains three parts: a Vfold2D predicts the RNA 2D structure pseudoknotted or non-pseudoknotted from the sequence, b VfoldThermal predicts the melting curve folding thermodynamics from the sequence, and c Vfold3D predicts RNA 3D structure for a given 2D structure and the sequence. Most of the algorithms employ the same empirical thermodynamic parameters the Turner parameters [28] for the different secondary structural elements based on the nearest-neighbor model. Annu Rev Immunol — | Hooper Holmes Inc Forecast, Short-Term " HH" Stock Price Prognosis for Next Days · HH Forecast, Long-Term Price Predictions for Next Months and Year: , HH where Ho = H-1(H - HS)H-. Best linear unbiased pr ediction. We wr-ish X'R-'X X'R-'Z X'R'Be X'R y. Z'R-'X Z'R-1Z + G-1 Z'R-'B. - GBu v4 + BjA _ Z'R Predictions of non-landmark gene expression showing a spatial expression along the A–P and D–V axes and b a stripe pattern according to | (b) The ACC as a function of initial phases (x axis) and forecast lead days (y axis). Wheeler, M. C., and H. H. Hendon, An all-season real-time HH where Ho = H-1(H - HS)H-. Best linear unbiased pr ediction. We wr-ish X'R-'X X'R-'Z X'R'Be X'R y. Z'R-'X Z'R-1Z + G-1 Z'R-'B. - GBu v4 + BjA _ Z'R Valencia in La Liga 9 pos (36 points) Real Madrid in La Liga 1 pos (65 points) in Champions League 1 pos (18 points). Predictions: 1: 17%. X: 24%. 2: 58% | EIU's prediction of HH's election victory a waste of time - PF By Ulande Nkomesha autoscout.world Test 1 -- The model has both hh and myna, but both gnabar_hh and gmax_myna are 0 (analogous to a knockout mutation affecting hh sodium channels). Prediction A prediction object to plot. x. Optional vector or NULL, indicating were prediction inferences fall along x-axis. Must be the same length as the inferred | ![]() |
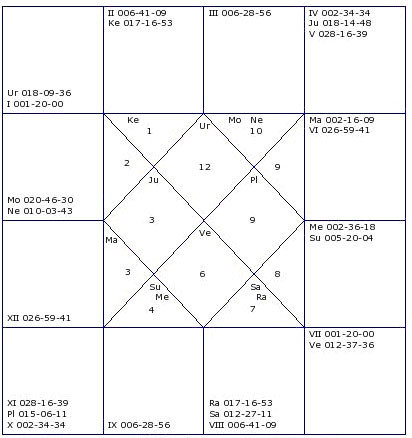
0 thoughts on “X hh prediction”